- Disruptive Technology Initiative
- / DTI Tech Tips
- / Smartphone digital phenotyping in older adults – what is neuropsychology’s role?
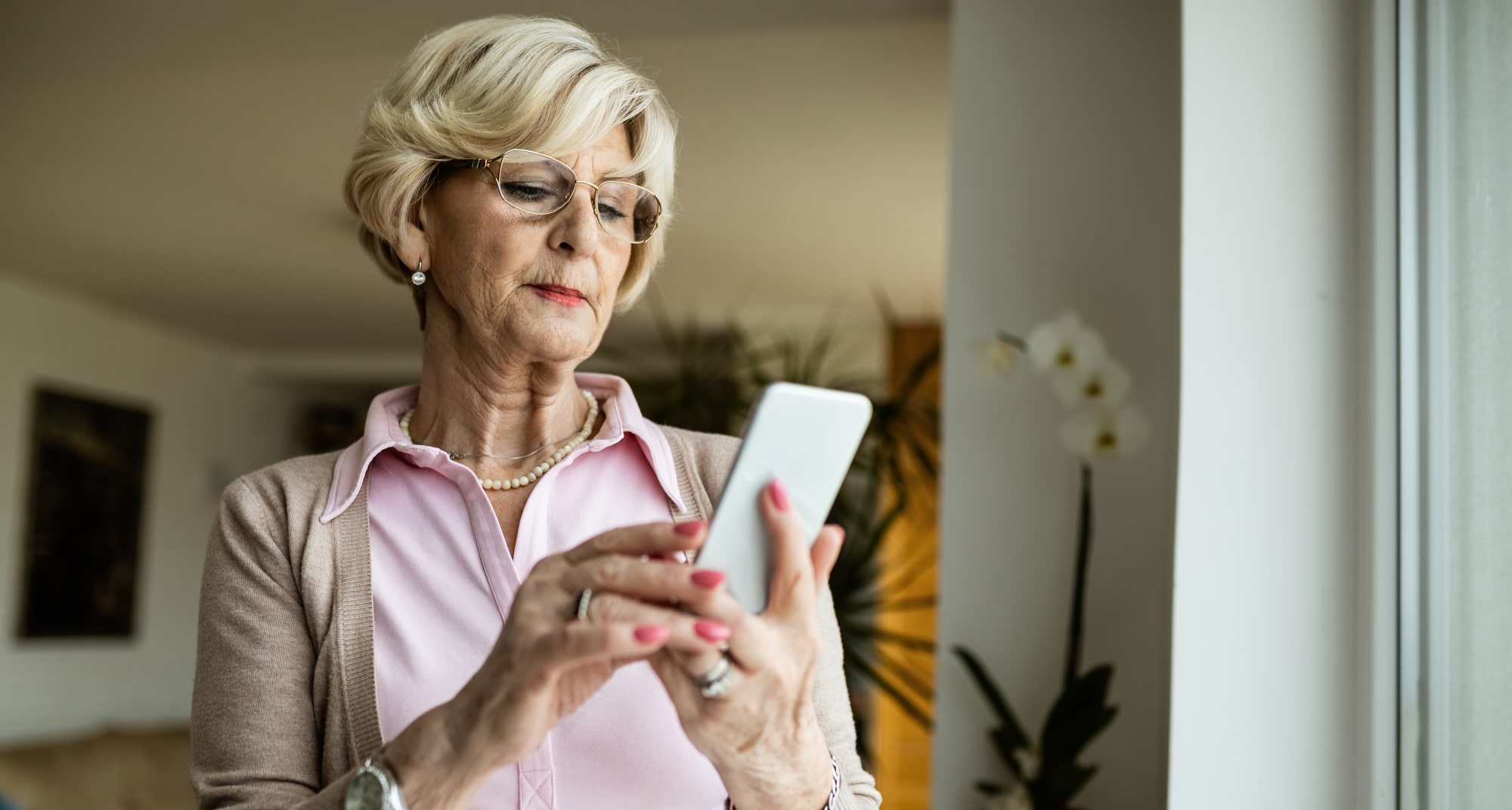
Smartphone digital phenotyping in older adults – what is neuropsychology’s role?
Author: Hackett, K.
Synopsis: Passively-collected sensor data from personal smartphones can provide complementary information about what happens outside the clinic, and may help us better understand how cognition, mood, and everyday activities are impacted by typical and atypical aging.
Author Disclosures: Nothing to disclose.
Overall Description: Smartphone digital phenotyping is one form of passive sensing that provides a rich array of real-world data that is passively captured by our smartphones. These data can be used to infer behavior, cognition and mood within the user’s natural environment. Preliminary support for this method has largely come from the field of psychiatry, where associations between various sensors and psychiatric outcomes such as depression and psychosis relapse have been identified. Specific sensors in most smartphones include a global positioning system (GPS) and accelerometer (to record location and movement trajectories, or estimate circadian routine and sleep). Device events are automatically captured including when and how often a phone is locked/unlocked and charged. Some phones allow collection of communication metadata which can be used as a proxy for socialization (how many texts/calls are sent/received). Keystroke dynamics (e.g., typing speed, interkey delay, deletions, autocorrects, pauses) can also be recorded by specific software and have shown relations with cognitive tests and diagnostic severity in those with mild cognitive impairment, Alzheimer’s disease, and multiple sclerosis.
How do we interpret these passive measures of everyday behavior in older adults? Conceptually, neuropsychologists are attuned to the mechanisms underlying changes that occur in healthy and pathological aging. Researchers and clinicians can and should reference established trends when interpreting digital phenotyping data in older adults. For example, expected declines in processing speed may manifest as slower typing and increased time spent using single applications. On the other hand, atypical difficulties with navigation that can occur in Alzheimer’s disease might be exhibited by increased irregularities in movement trajectories. Memory difficulties could be reflected by repetitive phone unlocking during a short timeframe, when someone needs to re-reference information they just viewed.
The Variability in Everyday Behavior (VIBE) model, based on the neuropsychological literature on aging and dementia, was developed to inform predictions of passive, everyday measures of activity and variability. For instance, the model predicts that decreased gait speed and increased gait variability observed in neurodegenerative processes may present as reduced range of movement and increased variability in GPS and accelerometer features from smartphones. Neuropsychologists have a key role to play in establishing theoretically-informed predictions and psychometric standards for this emerging method, to increase the chances that digital phenotyping data are clinically-relevant and reproducible.
Case Study Example: An ongoing study at Temple University was designed to establish preliminary validity for smartphone digital phenotyping among older adults. Hypotheses were informed by the VIBE model. In this study, participants with healthy cognition and mild cognitive impairment complete a baseline evaluation to collect validation metrics (comprehensive neuropsychological testing and questionnaires). The mindLAMP app – an open-source application for clinical and research use available on the App Store and Google Play – is downloaded onto participants’ personal smartphones. During the 4-week study period, mindLAMP passively, continuously and securely collects sensor data including GPS trajectories while participants go about their daily lives. At study completion, the app is removed and participants complete a series of debriefing questionnaires to assess their impressions. Preliminary, unpublished results show that participants are reporting comfort and satisfaction with study procedures, suggesting older adults may be open to sharing personal smartphone data when the methods, security details, and clinical relevance are clearly outlined. Preliminary analyses of GPS data suggest that greater levels of mobility (e.g., more distance traveled, less time spent in the home) are associated with better cognition and less depression, consistent with a priori hypotheses from the VIBE model. Inconsistent with hypotheses, greater GPS variability and less physical circadian routine were also associated with better cognition and less depression. Much work lies ahead before smartphone digital phenotyping can be used as a clinical tool. However, early studies are promising and will inform the next steps required to establish the validity and clinical utility of this method as well as novel conceptual frameworks to interpret results.
Helpful links:
- https://www.digitalpsych.org/lamp.html
- https://www.biaffect.com/
- Kourtis, L. C., Regele, O. B., Wright, J. M., & Jones, G. B. (2019). Digital biomarkers for Alzheimer’s disease: the mobile/wearable devices opportunity. NPJ digital medicine, 2(1), 9.
- Gordon, M. L., Gatys, L., Guestrin, C., Bigham, J. P., Trister, A., & Patel, K. (2019, May). App usage predicts cognitive ability in older adults. In Proceedings of the 2019 CHI Conference on Human Factors in Computing Systems (pp. 1-12).
- Chen, R., Jankovic, F., Marinsek, N., Foschini, L., Kourtis, L., Signorini, A., … & Trister, A. (2019, July). Developing measures of cognitive impairment in the real world from consumer-grade multimodal sensor streams. In Proceedings of the 25th ACM SIGKDD international conference on knowledge discovery & data mining (pp. 2145-2155).
- Chen, M. H., Leow, A., Ross, M. K., DeLuca, J., Chiaravalloti, N., Costa, S. L., … & Demos, A. P. (2022). Associations between smartphone keystroke dynamics and cognition in MS. Digital Health, 8, 20552076221143234.
- Hackett, K., & Giovannetti, T. (2022). Capturing Cognitive Aging in Vivo: Application of a Neuropsychological Framework for Emerging Digital Tools. JMIR aging, 5(3), e38130.
Limitations: There are many interpretive challenges to this method that require further investigation. For example, the extent to which individual differences (e.g., phone type, phone use habits, demographic variables) impact relationships between sensor data and cognition is still unknown. We also do not know how much sensor data are necessary to make clinically-meaningful predictions. Logistically, the collection of high-frequency sensor data can sometimes lead to battery drain, which is both cumbersome to the patient and disrupts the naturalistic aspect of this approach. Ideally, this issue will resolve with advances in smartphone battery technology, but may also be addressed by cutting back on sampling frequency. Finally, issues related to data privacy and security are extremely important to consider and remain an evolving target as regulatory guidelines continue to shift.
Justice, Equity, Diversity, Inclusion Issues: Key to the success and generalizability of the digital phenotyping approach is the recruitment of diverse cohorts in validation studies. The case study reported here included a majority non-Hispanic White, highly educated and urban sample, which should be considered a major limitation. Future studies will need to focus on recruiting more diverse participants (e.g., across race, ethnicity, gender, socioeconomic status, spoken language, educational attainment, and geographic region). Because of increased affordability and rates of smartphone ownership among diverse populations around the world, this method holds promise to address gaps in accessibility and objectivity that are present in many of our existing diagnostic tools.
Glossary:
- GPS: A Global Positioning System (GPS) provides location information based on signals it receives from satellites. GPS sensors within smartphones can tell us the approximate latitude, longitude, and altitude of the smartphone, which provides a proxy for the user’s location.
- Accelerometer: A motion sensor within smartphones that can be used to detect what type of activity the user is doing. The accelerometer captures the X-Y-Z coordinates of the phone in G-forces, which provides information on the phone’s orientation and acceleration in space.
- Metadata: Data that provides information about other data, but not the content of the data. For example, communication metadata provides the number of texts/calls sent/received from unique phone numbers along with the associated timestamp, but would not provide the content of the texts/calls or the sending/receiving phone number.
- Variability in Everyday Behavior (VIBE) model: A conceptual framework proposed by Hackett & Giovannetti (2022) that integrates known trends from the cognitive aging literature on changes in activity level and intraindividual variability; this model provides testable hypotheses and a theoretical framework for digital phenotyping studies that collect information on everyday behavior in older adults.
- mindLAMP app: An open-source smartphone application developed for research and clinical use by the Division of Digital Psychiatry at the Beth Israel Deaconess Medical Center. mindLAMP can be used to collect a range of active (e.g. survey, mobile cognitive testing) and passive (sensor) data.
- Physical circadian routine: A mobility feature that can be derived from GPS data, which compares the similarity in a person’s locations over the course of a day to that of the other days in the data set. The value ranges between 0 and 1, with 1=identical routine and 0=completely different routine.
Comments/Experiences to Share?
Dear AACN members, please log in to share your comments or questions here.