- Disruptive Technology Initiative
- / DTI Tech Tips
- / Passive sensing: what do our devices say about our brain and behavior?
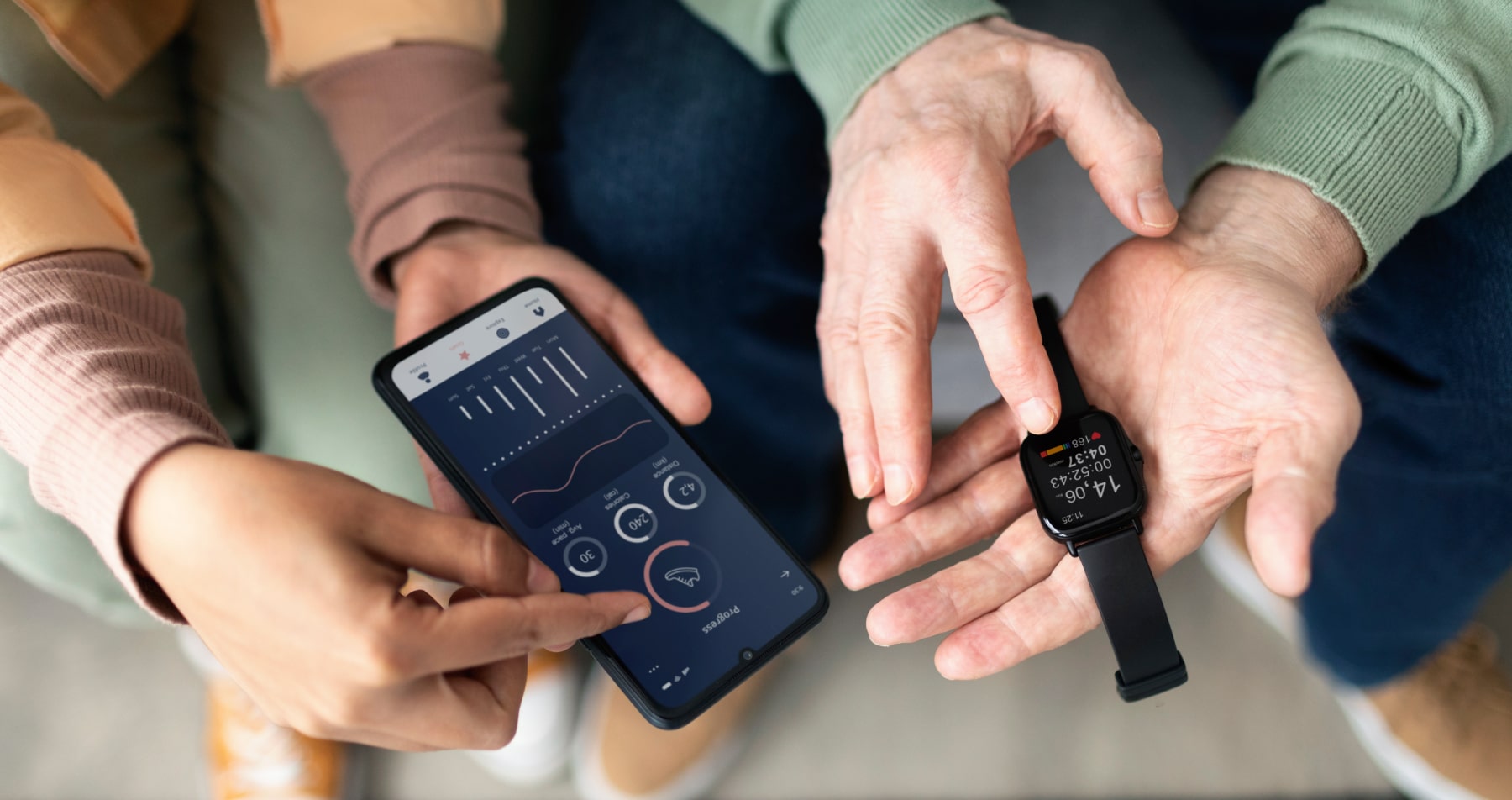
Passive sensing: what do our devices say about our brain and behavior?
Author: Chen, M. H.
Author disclosure: Dr. Chen currently conducts passive sensing research using wearable devices, which is funded by Rutgers University.
Synopsis: Unobtrusive sensing approaches, such as mobile devices and in-home sensors, enable continuous data collection of everyday life states/behaviors and do not require users to have high technological literacy.
Overall description and clinical examples: Digital phenotyping is a method of inferring human states and behaviors through digital devices. The most common digital device is the smartphone, which is owned by 85% of Americans (Pew Research Center, 2021). Smartphones have become a vehicle for brief real-world cognitive assessments. Besides active tasks and surveys, smartphones contain sensors that can elucidate movement/motor functioning, location, ambient light social engagement, mood, and cognition. For example, we may use a smartphone to monitor onset of a depressive episode based on movement, location, and call/text data (e.g., increase in time spent in the home, less contact with friends and family). Changes in cognitive and motor functioning may be inferred from changes in an individual’s typing patterns on their phones. Besides smartphones, smartwatches (e.g., Apple Watch, Fitbit) have become increasingly ubiquitous. Not only can smartwatches be used to track physical activity and sleep, they can also gather physiological information such as heart rate and skin conductance, which may elucidate various mental states (e.g., stress, depression, anxiety, fatigue).
In addition to personal devices, there are various sensors that can be placed in our daily environment such as our homes and cars. Sensors in the home can track movement between rooms, sleep/wake patterns, and medication adherence (e.g., digital pillbox). Researchers have begun to study these home-based multimodal sensors in “smart homes,” which have the potential to track real-world functional decline among elderly individuals and individuals with dementia (e.g., falls, decreased activity). Further, modern automobiles contain computers and sensors, from which driving patterns can be characterized. Given that driving is a cognitively demanding behavior, decline in driving may be an early sign of cognitive decline that is not only ecologically valid but may be more sensitive than traditional neuropsychological tests. Voice and speech changes are another set of early markers of cognitive decline and may be recorded via sensors and analyzed using acoustic and natural language processing techniques. Mobile or environmental sensors can facilitate personalized just-in-time adaptive interventions, which are interventions delivered at the moment when the person needs it the most (e.g., reminder about increasing physical activity after long sedentary period detected, deliver a mindfulness intervention when detecting stress and anxiety through physiological sensors, call emergency services when a fall is detected in the home).
The methods outlined in this article are all sensing modalities that can be deployed passively within the subject’s natural environment, which may be more universally accepted among individuals with various levels of technological literacy and different linguistic and cultural backgrounds. Compliance is less problematic since users are not required to do anything out of the ordinary. Moreover, these sensing methods enable continuous data collection which may be used to develop machine learning algorithms that can integrate multiple streams of data that violate traditional statistical assumptions (e.g., normality).
Justice, Equity, Diversity, Inclusion Issues: Passive sensing modalities have the potential to be more inclusive than more active assessment methods mediated by novel digital technology (e.g., smartphone-based cognitive assessment) because they do not require a certain level of technological proficiency and can be language-independent. However, some of the devices included in this article and/or stable Internet access to transmit the data may not be financially feasible for everyone, especially those in low- and middle-income countries. Even within the U.S., black users have been found to have higher rates of data non-collection than other races (could be due to differences in hardware, software, Internet connectivity, etc.)3. Some technologies may not work universally across diagnostic groups. For example, step count algorithms in smart watches are inappropriate for wheelchair users. Thus, besides market forces which may continue to make these devices cheaper and more accessible, analytic adjustments (e.g., device-specific cutoffs, imputation for missing data) may be needed to render a more equitable conclusion across groups when using these technologies.
Limitations: Privacy is always a significant issue to address with remote monitoring approaches since the data are not collected in a controlled confidential environment. However, there are safeguards for protecting the patient’s/participant’s confidentiality, such as limiting the amount of identifying information that is collected (e.g., use subject ID as identifier) and ensuring adequate encryption of data when stored on a device and/or transmitted to a cloud-based storage system. Nevertheless, due to the novelty of this type of data, there is a lack of guidelines and frameworks from regulatory bodies about what is considered identifying information (e.g., are typing patterns or locations considered identifying?) and how to best protect it. When data are collected using private software, ownership of data needs to be clarified (i.e., is it the company, the clinician/researcher who prescribed it, or the patient/participant who owns the data), as it would affect what happens to the data beyond immediate clinical use (e.g., data being sold to third parties). Informed consent needs to be clear to the patient/participant about what information will be collected and how the data will be used and shared. While the ability to collect high-frequency data is a strength of passive sensing technologies, it may also be a limitation as this type of data requires more sophisticated data science analysis techniques (e.g., machine learning).
Helpful links to further reading:
- Insel, T. R. (2017). Digital phenotyping: technology for a new science of behavior. Jama, 318(13), 1215-1216.
- Kourtis, L. C., Regele, O. B., Wright, J. M., & Jones, G. B. (2019). Digital biomarkers for Alzheimer’s disease: the mobile/wearable devices opportunity. NPJ digital medicine, 2(1), 1-9.
- Kiang, M. V., Chen, J. T., Krieger, N., Buckee, C. O., Alexander, M. J., Baker, J. T., … & Onnela, J. P. (2021). Sociodemographic characteristics of missing data in digital phenotyping. Scientific reports, 11(1), 1-11.
- mindLAMP: open-source software platform created by Dr. John Torous’s team at Beth Israel Deaconess Medical Center. https://www.digitalpsych.org/lamp.html
- MD2K: open-source software platform from the Center of Excellence for Mobile Sensor Data-to-Knowledge, one of the national Big Data Centers of Excellence awarded by the NIH, including investigators from 13 universities. https://archive.md2k.org/software.html
Glossary:
- Digital phenotyping: a method of inferring human states and behaviors through digital devices such as smartphones and smartwatches. Digital phenotyping can involve both active tasks (e.g., cognitive tasks, surveys) and passive sensing (e.g., physical activity, sleep).
- Natural language processing: using computers to analyze text data in order to characterize or make predictions (e.g., identifying spam emails, chat bots on business websites).
- Just-in-time adaptive interventions: interventions that are delivered at the moment when the person needs it the most; sensors may be used as inputs to facilitate these interventions (e.g., smartwatches can tell the user to get up and walk around after detecting a sedentary period).
- Machine learning: using computers to learn information and make predictions; can involve supervised (when the true information is known; e.g., regression-based methods) or unsupervised learning (when the true information is unknown and the computer has to find the pattern; e.g., cluster analysis). Machine learning algorithms are not constrained by traditional statistical assumptions such as normality and homoscedasticity. They are also ideal when dealing with a large amount of data (i.e., “big data”) from different sources.
Comments/Experiences to Share?
Dear AACN members, please log in to share your comments or questions here.