- Disruptive Technology Initiative
- / DTI Tech Tips
- / Artificial Intelligence in Neuropsychology: The Promise of Reinforcement Learning
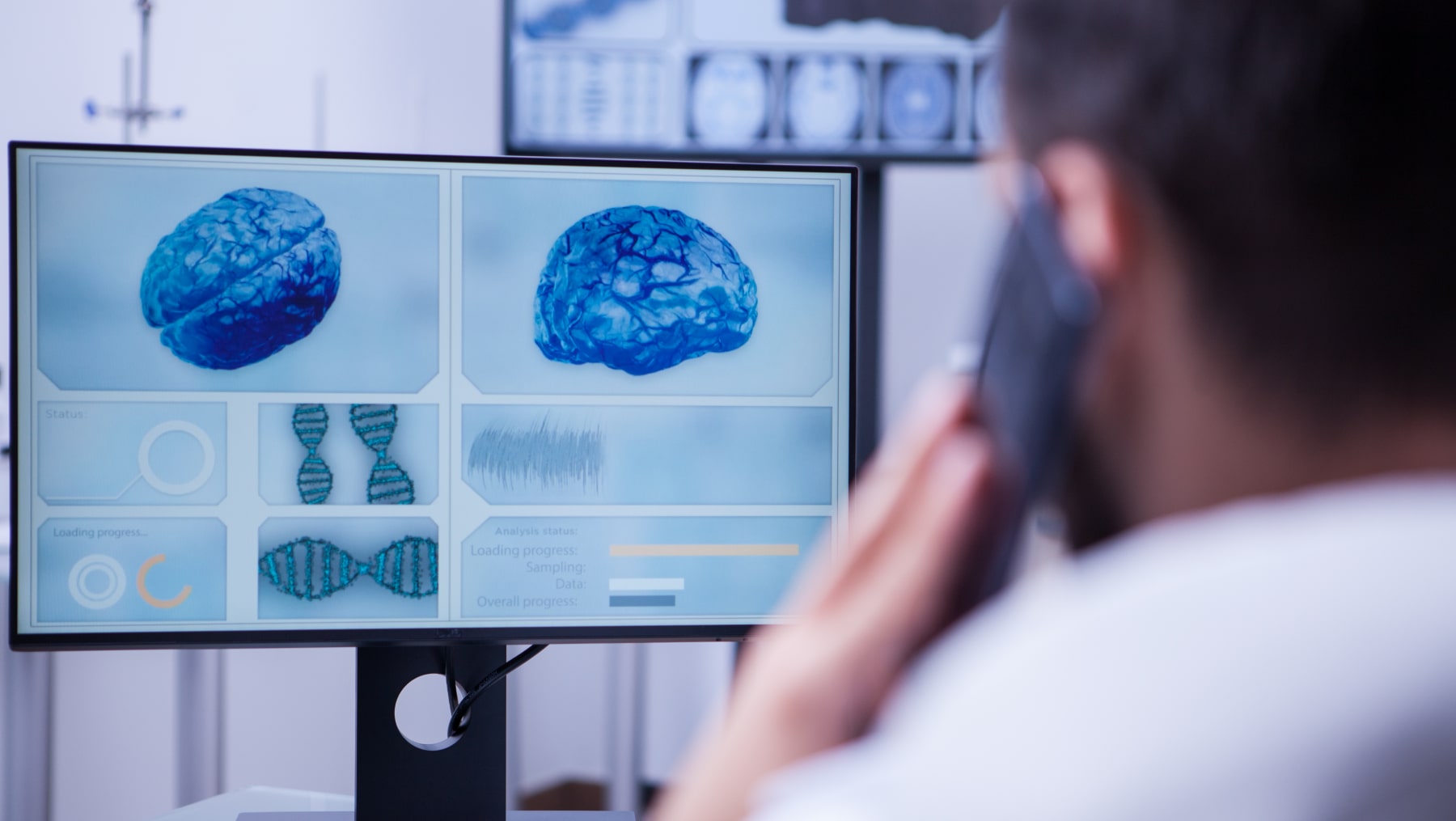
Artificial Intelligence in Neuropsychology: The Promise of Reinforcement Learning
Author: Wolff, B.
Author Disclosures: Nothing to disclose.
Synopsis. Artificial intelligence (AI) is a process in which complex information is intelligently analyzed, enabling machines to explore, learn, discover, and reason from knowledge. AI has the potential to modernize clinical neuropsychology, and there is great promise in fully deployable AI tools in early diagnosis, management, and treatment of brain-based conditions.
Overall Description. AI is an umbrella term referring to the building of systems or machines to accomplish tasks which would otherwise require human intelligence, such as decision making. AI can assist us in making better clinical decisions and replacing human judgment in some functional areas (e.g., neuroimaging). AI can unlock hidden information in big data to inform our practice, reduce diagnostic and therapeutic errors in clinical practice, and make real-time inferences for health risks and outcome prediction. AI can also extract phenotypic features from case reports to enhance diagnostic accuracy, and enable precision medicine. Efficient analysis of data could allow triaging where AI software ranks patients in order or priority or removes them from lists. This may also reduce clinician burnout, with AI performing more time-consuming tasks. AI use in scoring could save clinicians time better spent helping patients directly (e.g., automated scoring of thousands of RCFT and clock drawings).
AI virtual and robotic agents optimize reinforcement learning through use of algorithms that respond independently of human guidance through a virtually embodied presence. Embodied AI has the promise of improving quality of care and reducing costs, while also reaching vulnerable or remotely located populations. With expertise in brain-behavior relationships and psychometrics, clinical neuropsychologists are well equipped to lead the AI revolution and develop new tools to solidify the importance of our field. This will involve thinking outside of the box about how our skills can be integrated with the evolution of technology.
Case Study Examples. AI has been used in stroke cases, including early disease prediction and diagnosis, treatment, and outcome prediction and prognosis evaluation. Wearable devices analyze normal versus pathological gaits for stoke prediction, while machine learning (ML) alerts physicians to onset of stroke. ML applied to neuroimaging data correctly identifies endophenotypes of motor disability after stroke, identifies stroke lesions on MRI, and analyzes performance of stroke treatment. In aphasia diagnosis and management, AI has been implemented in assessment, therapy, self-management and discovery of novel treatment avenues. For instance, Constant therapy for self-management when clinician input is not available. Deep-learning AI models and programs such as ChatGPT can also reliably distinguish individuals with Alzheimer’s disease from healthy controls using speech data.
Embodied AI supports a range of emotional, cognitive and social processes. Chatbots (e.g., Tess, Sara, Wysa, Woebot) can treat depression and anxiety, engaging with the patient and detecting emotional and cognitive symptoms via learning and natural language processing (NLP). Avatars, such as the Avatar Project (computer-generated faces on screens), are used to treat psychosis patients, and also enhance medication adherence. Avatars such as Kognito are used in risk prevention education to help students identify risk situations, while virtual patients provide students with life-like clinical interview practice. AI animal-like robots such as Paro are used for patients with dementia as at-home health care assistants, responding to speech and movement with ‘dialog’. Socially assistive robotics (RoboTherapy, Nao) help children with autism improve social skills, facial recognition and joint attention.
Limitations. There are no standards to assess the safety and efficacy of AI. There are patient privacy, information and safety concerns due to risks with portable and cloud-enabled data storage and the possibility of data breaches. Behavioral observations are also yet to be solidified into AI; the highly anxious patient presenting with an amnestic memory profile might not be classified by AI under an accurate phenotype. There are ethical dilemmas, such as when a chatbot identifies a patient as high suicide or stroke risk with no local services available. AI will need rigorous risk assessments prior to clinical use (refer to the ‘AI4People Framework’).
Justice, Equity, Diversion, Inclusion. AI can reach populations which may be less accessible via traditional healthcare routes, by providing low-threshold, therapeutic interventions via chatbots or avatars to people in resource-poor settings, and AI assessment for people in remote areas without on-site neuropsychological services. This may also apply in higher income locations for people without insurance. Low-threshold interventions conducted in patients’ own homes or via portable methods may be suitable options. AI could also act as an entry point for traditional assessment and intervention. This relies on access to a technology platform (i.e., smartphone), which excludes 16% of the world’s population.
There is risk of physician bias with AI interpretation, and models based only on electronic health record data are also likely to be biased, as the AI is missing information about everyday dynamic functioning. AI is less likely to understand the biopsychosocial embedded systems within which the patient developed; integration of AI into healthcare practices must be responsive to evolving understandings of the role of technology in society and culture.
Helpful Links
Machine learning (ML) is one branch of AI (see our ML Tip for more). Wearable AI can be used to develop diagnostic and surveillance models (see our Passive Data Tip and Smartphone Phenotyping Tip for more). Virtual reality AI is used in diagnostic and treatment modalities (see our Virtual Reality Tip for more). The value of AI could be enhanced on a larger scale by aggregating ‘big’ data (i.e., volume, velocity, variety) into collaborative repositories to improve the accuracy of AI, such as linking multi-modal data sources to identify precision biomarkers associated with cognitive and psychiatric phenotypes (see our CDE Tip for more).
Other helpful articles on this topic:
Abd-Alrazaq, A., Alhuwail, D., Schneider, J., Toro, C. T., Ahmed, A., Alzubaidi, M., Alajlani, M., & Househ, M. (2022). The performance of artificial intelligence-driven technologies in diagnosing mental disorders: an umbrella review. NPJ digital medicine, 5(1), 87. https://doi.org/10.1038/s41746-022-00631-8
Adikari, A., Hernandez, N., Alahakoon, D., Rose, M. L., & Pierce, J. E. (2023). From concept to practice: A scoping review of the application of AI to aphasia diagnosis and management. Disability and Rehabilitation, 0(0), 1–10. https://doi.org/10.1080/09638288.2023.2199463
Battista, P., Salvatore, C., Berlingeri, M., Cerasa, A., & Castiglioni, I. (2020). Artificial intelligence and neuropsychological measures: The case of Alzheimer’s disease. Neuroscience and biobehavioral reviews, 114, 211–228. https://doi.org/10.1016/j.neubiorev.2020.04.026
Fiske, A., Henningsen, P., & Buyx, A. (2019). Your Robot Therapist Will See You Now: Ethical Implications of Embodied Artificial Intelligence in Psychiatry, Psychology, and Psychotherapy. Journal of Medical Internet Research, 21(5), e13216. https://doi.org/10.2196/13216
Floridi, L., Cowls, J., Beltrametti, M., Chatila, R., Chazerand, P., Dignum, V., Luetge, C., Madelin, R., Pagallo, U., Rossi, F., Schafer, B., Valcke, P., & Vayena, E. (2018). AI4People-An Ethical Framework for a Good AI Society: Opportunities, Risks, Principles, and Recommendations. Minds and Machines, 28(4), 689–707. https://doi.org/10.1007/s11023-018-9482-5
Jiang, F., Jiang, Y., Zhi, H., Dong, Y., Li, H., Ma, S., Wang, Y., Dong, Q., Shen, H., & Wang, Y. (2017). Artificial intelligence in healthcare: Past, present and future. Stroke and Vascular Neurology, 2(4). https://doi.org/10.1136/svn-2017-000101
Kang, M. J., Kim, S. Y., Na, D. L., Kim, B. C., Yang, D. W., Kim, E. J., Na, H. R., Han, H. J., Lee, J. H., Kim, J. H., Park, K. H., Park, K. W., Han, S. H., Kim, S. Y., Yoon, S. J., Yoon, B., Seo, S. W., Moon, S. Y., Yang, Y., Shim, Y. S., … Youn, Y. C. (2019). Prediction of cognitive impairment via deep learning trained with multi-center neuropsychological test data. BMC medical informatics and decision making, 19(1), 231. https://doi.org/10.1186/s12911-019-0974-x
Kashyap, K., & Siddiqi, M. I. (2021). Recent trends in artificial intelligence-driven identification and development of anti-neurodegenerative therapeutic agents. Molecular diversity, 25(3), 1517–1539. https://doi.org/10.1007/s11030-021-10274-8
Miller J. B. (2019). Big data and biomedical informatics: Preparing for the modernization of clinical neuropsychology. The Clinical neuropsychologist, 33(2), 287–304. https://doi.org/10.1080/13854046.2018.1523466
Parsons, T. D., & Duffield, T. (2019). National Institutes of Health initiatives for advancing scientific developments in clinical neuropsychology. The Clinical neuropsychologist, 33(2), 246–270. https://doi.org/10.1080/13854046.2018.1523465
Comments/Experiences to Share?
Dear AACN members, please log in to share your comments or questions here.